The Role of Training Data for Self Driving Cars in Transforming Mobility
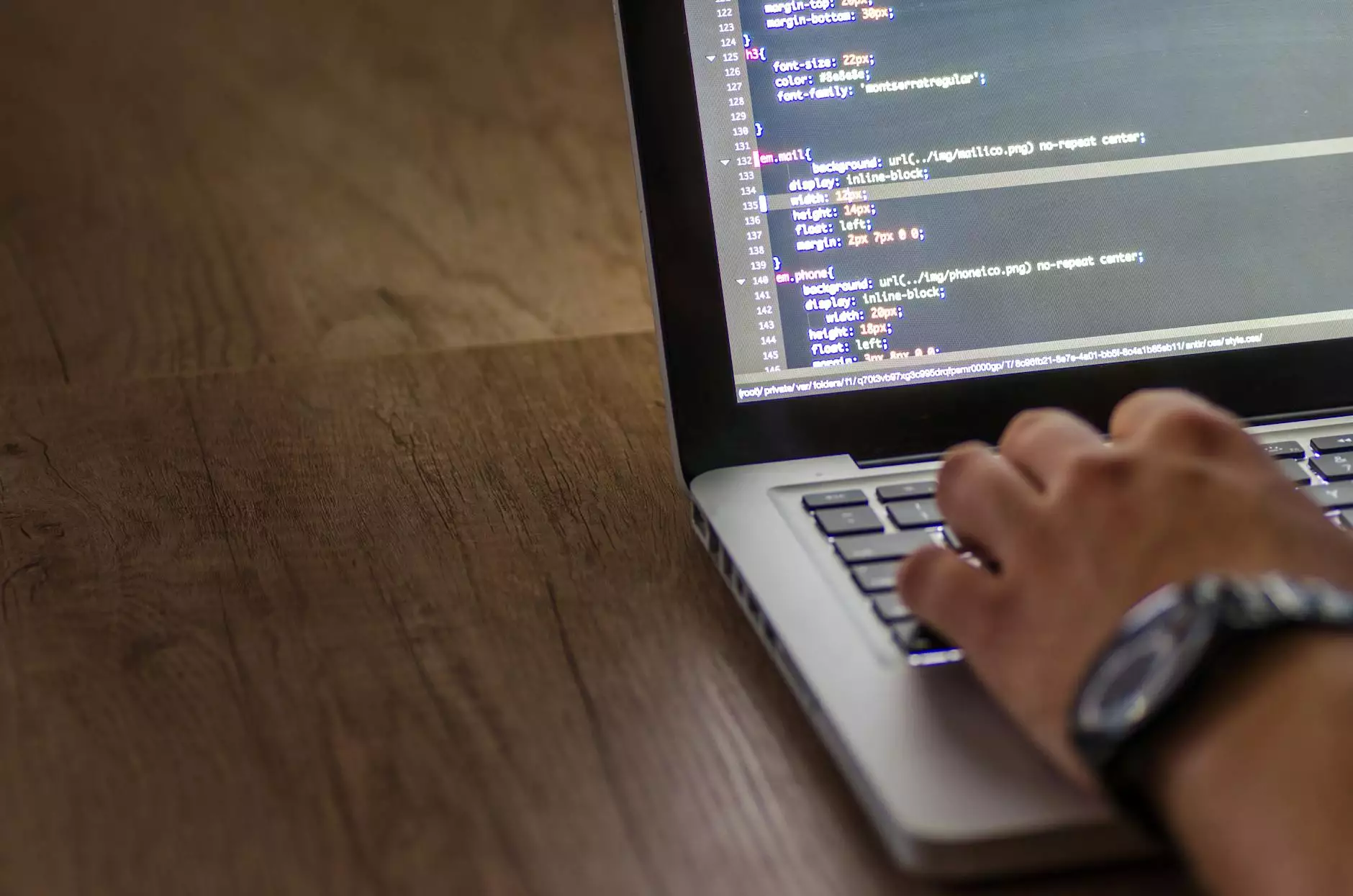
In recent years, the automotive industry has witnessed a remarkable shift towards the development of self-driving cars. This significant technological advancement is primarily driven by the need for improved safety, efficiency, and convenience in transportation. At the heart of this revolution lies the concept of training data for self driving cars, which plays a crucial role in enabling these vehicles to navigate the roads safely and reliably.
Understanding Self-Driving Cars
Self-driving cars, also known as autonomous vehicles, utilize a combination of hardware and software to operate without human intervention. They rely on a sophisticated array of technologies including sensors, cameras, artificial intelligence (AI), and machine learning to interpret their surroundings and make decisions in real-time.
The Importance of Training Data
The backbone of any intelligent system is data. In the case of self-driving cars, training data is vital for several reasons:
- Machine Learning Models: Machine learning algorithms require vast amounts of data to learn from. Training data for self driving cars includes miles of driving footage under various conditions to train these models effectively.
- Scenario Simulation: Real-world driving is filled with unpredictable events. Training data helps simulate myriad scenarios to ensure the vehicle can handle everything from typical traffic patterns to unexpected obstacles.
- Continuous Improvement: As self-driving technology evolves, so does the data it relies on. New data helps refine algorithms, enabling cars to become smarter and more capable over time.
Types of Training Data for Self Driving Cars
Training data can be categorized into various formats and sources, each serving distinct purposes in the development of autonomous vehicle technology.
1. Real-World Driving Data
This data is collected from vehicles operating on actual roads. It captures diverse driving conditions such as:
- Different weather conditions (rain, snow, fog)
- Varied road types (urban, rural, highways)
- Traffic scenarios (heavy traffic, free-flowing traffic)
2. Synthetic Data
Advanced simulations are used to generate synthetic datasets. This type of data provides valuable training scenarios that may be rare or dangerous to collect in real life, such as:
- Unexpected pedestrian crossings
- Accidents involving other vehicles
- Animals on the road
3. Labeled Data
For machine learning models to learn, they need labeled data — information categorized in a way that the AI can understand. Examples include:
- Classifying objects (cars, pedestrians, bicycles)
- Labeling road signs and traffic signals
- Mapping lane markings and boundaries
The Process of Collecting Training Data
Collecting training data for self driving cars is a multifaceted process that includes several stages:
Data Capture
Initially, data is captured using onboard sensors and cameras installed in test vehicles. These devices gather information continuously while the vehicle is in motion, recording a wide range of scenarios.
Data Annotation
Raw data collected from vehicles is not immediately useful. It must be annotated and labeled by trained professionals or through automated processes. This step ensures that each piece of data is categorized accurately for machine learning models to process.
Data Validation
Once annotated, the data undergoes a validation process to ensure its quality and reliability. Errors in labeling can lead to incorrect model training, resulting in unsafe driving behaviors.
Use Cases of Training Data in Autonomous Driving Technology
Training data for self driving cars supports a broad range of applications within the domain of autonomous driving. Here are some significant use cases:
Enhanced Safety Features
By analyzing vast amounts of training data, manufacturers can develop improved safety features such as:
- Automatic emergency braking systems
- Lane-keeping assistance
- Adaptive cruise control
Navigation and Route Planning
Training data contributes to sophisticated navigation algorithms that help vehicles process real-time traffic data, optimizing routes based on current conditions and predicted scenarios.
User Experience Improvements
Data can also be utilized to enhance rider experience, by learning user preferences and adjusting driving styles accordingly.
Challenges in Gathering Training Data
Despite the importance of training data, there are significant challenges in its collection and utilization:
Data Privacy Concerns
As vehicles gather data about their surroundings, concerns arise regarding user privacy and data protection. Manufacturers must implement stringent measures to ensure that sensitive information is kept secure.
Data Bias and Representation
A diverse dataset is essential to prevent model bias. If the training data does not represent all driving conditions and scenarios, it can lead to poor performance in real-world applications.
Cost of Data Annotation
The process of data annotation can be labor-intensive and costly. Companies must balance the need for high-quality data with budget constraints.
The Future of Training Data for Self Driving Cars
The future of autonomous vehicles relies heavily on the continued evolution of training data. As technology advances, we can expect the following trends:
Integration of AI
Artificial intelligence will play a critical role in both the generation and processing of training data, making it easier to derive insights and enhance vehicle performance.
Collaboration Across Industries
Collaboration between automotive, technology, and data industries will lead to richer datasets, leading to safer and more efficient self-driving solutions.
Regulatory Frameworks
Governments will likely implement guidelines and standards for data collection and usage, ensuring safety and privacy for autonomous vehicle users.
Conclusion
The advancement of self-driving cars heralds a new era in transportation, primarily driven by the necessity for comprehensive and high-quality training data for self driving cars. As we embrace this technological revolution, it is imperative to focus on gathering diverse data, ensuring proper annotation, and navigating the associated challenges. The road ahead for autonomous vehicles gleams with promise, revolutionizing the way we think about mobility and safety on our roads.