The Importance of Machine Learning Data Labeling: Enhancing Your AI Models
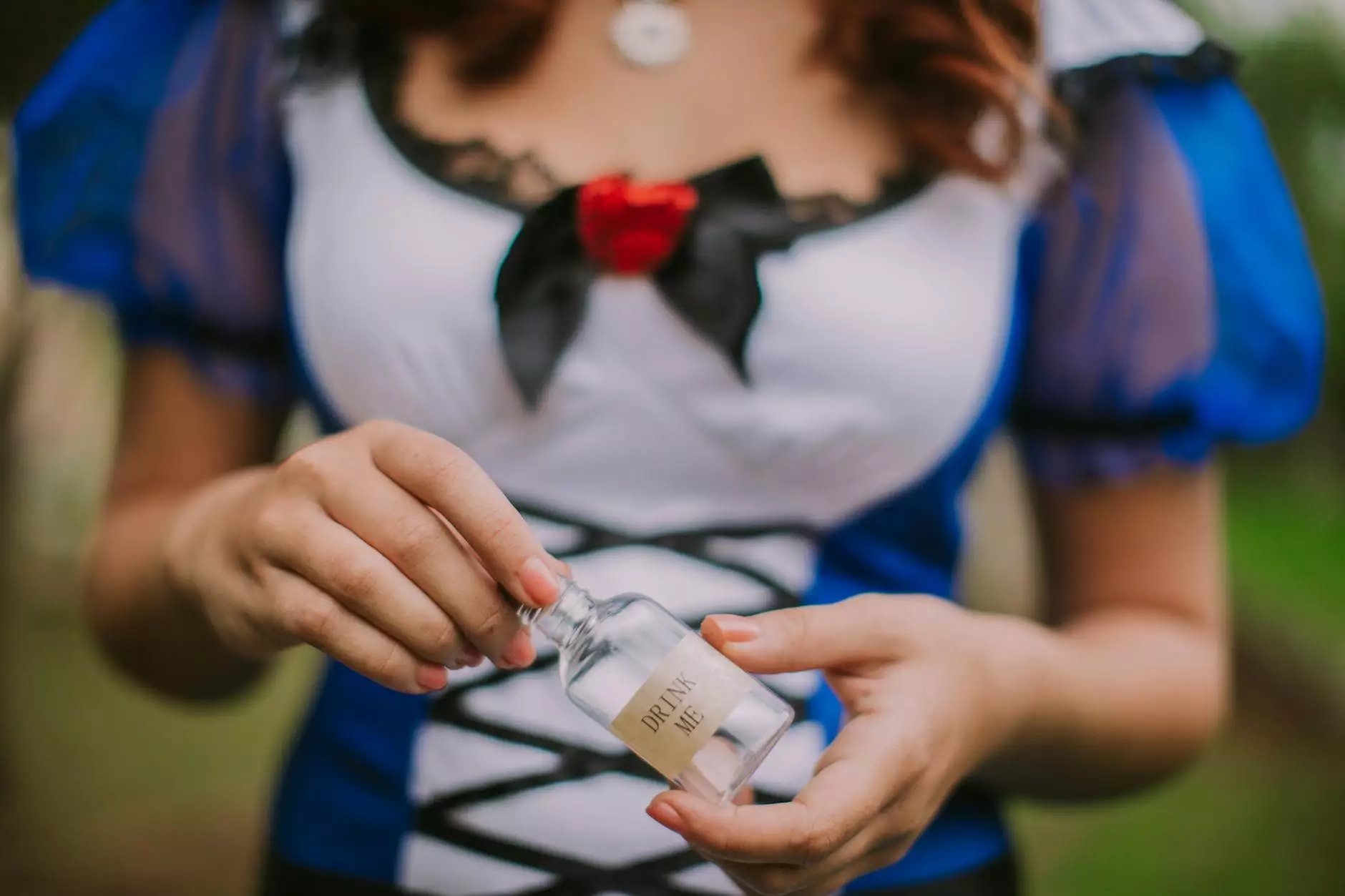
In today's digital age, businesses and organizations are increasingly seeking innovative solutions driven by artificial intelligence (AI). At the core of successful AI implementations lies an essential component: machine learning data labeling. This process involves annotating data to make it understandable and usable for machine learning models. As the complexity of AI grows, so does the need for efficient and accurate data labeling solutions.
Understanding Machine Learning Data Labeling
Machine learning data labeling refers to the practice of tagging or annotating data—such as images, texts, or audio files—so that machine learning algorithms can learn from it. The labeled data serves as a training set, where the model identifies patterns and makes predictions based on the provided information. Without proper labeling, machine learning models may struggle to learn effectively, leading to inaccurate outcomes.
Why is Data Labeling Crucial for Successful AI Models?
The effectiveness of any machine learning model is heavily reliant on the quality of the training data. Here are a few reasons why machine learning data labeling is so crucial:
- Improves Accuracy: Properly labeled data enhances the model's ability to make accurate predictions and classifications.
- Supports Different Learning Types: Various types of machine learning, such as supervised, unsupervised, and reinforcement learning, depend on quality data labeling.
- Enables Complex Analysis: Labeled data facilitates better analysis, allowing businesses to derive valuable insights from their models.
- Bespoke Solutions: Custom labeling can cater to specific industry needs, making the model highly relevant to particular markets.
The Data Annotation Process
The journey of machine learning data labeling typically involves several steps:
1. Data Collection
First, raw data is gathered from various sources, including databases, web scraping, or user-generated content. This step is crucial, as diverse datasets enhance the robustness of the model.
2. Preprocessing
Once collected, data must be cleaned and refined. This process includes removing duplicates, correcting errors, and converting data into a suitable format for annotation.
3. Annotation
This is where the actual labeling happens. Annotators assign tags, labels, or other metadata to the data. This step can be done manually or through automation, depending on the scale of the project.
4. Quality Assurance
After annotation, it's essential to perform quality checks to ensure the accuracy and consistency of the labeled data. This can involve reviews by additional experts or automated checks.
5. Model Training
With high-quality labeled data, machine learning models can now be trained to learn from this input, improving their performance over time.
Choosing the Right Data Annotation Tool or Platform
When it comes to machine learning data labeling, selecting a robust data annotation tool or platform is vital. Below are some key considerations when choosing the right one:
1. Scalability
As businesses grow, the volume of data often increases. Your chosen tool should handle large datasets seamlessly without compromising performance.
2. User-Friendliness
The platform should be easy to navigate, allowing team members, regardless of their technical background, to contribute efficiently to the labeling process.
3. Flexibility
Different projects have unique needs. A good data annotation tool should support various data types (images, videos, texts) and allow for custom labeling options.
4. Integration Capabilities
Ensure that the tool can easily integrate with existing data pipelines and machine learning frameworks to streamline the entire process.
5. Cost-Effectiveness
Consider your budget while evaluating data annotation solutions. Choose a platform that offers a balance between features and affordability.
The Future of Data Annotation and Its Role in AI
The landscape of machine learning data labeling is evolving rapidly. Emerging technologies such as automated labeling tools and advanced algorithms are making the process faster and more efficient. Here’s what the future holds:
1. Increased Automation
With advancements in AI, automated data labeling systems are becoming more common. These systems can significantly reduce the time and cost associated with manual annotation.
2. Crowdsourced Labeling
Involving a large pool of contributors can enhance the diversity and quality of labeled data. Crowdsourcing platforms are increasingly being used to gather annotations from people worldwide.
3. Enhanced Collaboration
Tools that promote real-time collaboration among team members, regardless of geographical location, will continue to be essential as remote work becomes more prevalent.
4. Focus on Quality
The emphasis on quality assurance will only grow. Businesses must invest in strategies and technologies that ensure high-quality labeled datasets for their models.
Conclusion
In conclusion, machine learning data labeling plays a pivotal role in the success of AI initiatives across industries. By investing time and resources into developing high-quality labeled datasets, businesses can unlock the full potential of their machine learning models. The right data annotation tools and platforms are crucial in this journey, supporting efficiency, quality, and scalability. As the field advances, staying informed about new technologies and trends in data labeling will empower organizations to remain competitive in an increasingly data-driven world.
Your Next Steps
Are you ready to enhance your AI projects with expert machine learning data labeling? Explore keylabs.ai for advanced data annotation tools and platforms that can transform your machine learning initiatives. Invest in Quality Data, Invest in Your Future!